Institute for Genomic Statistics and Bioinformatics
Welcome to IGSB
We are convinced that precision medicine can only be achieved when human and artificial intelligence join efforts. Please have a look at our research and get in contact if you have any questions or would like to collaborate!
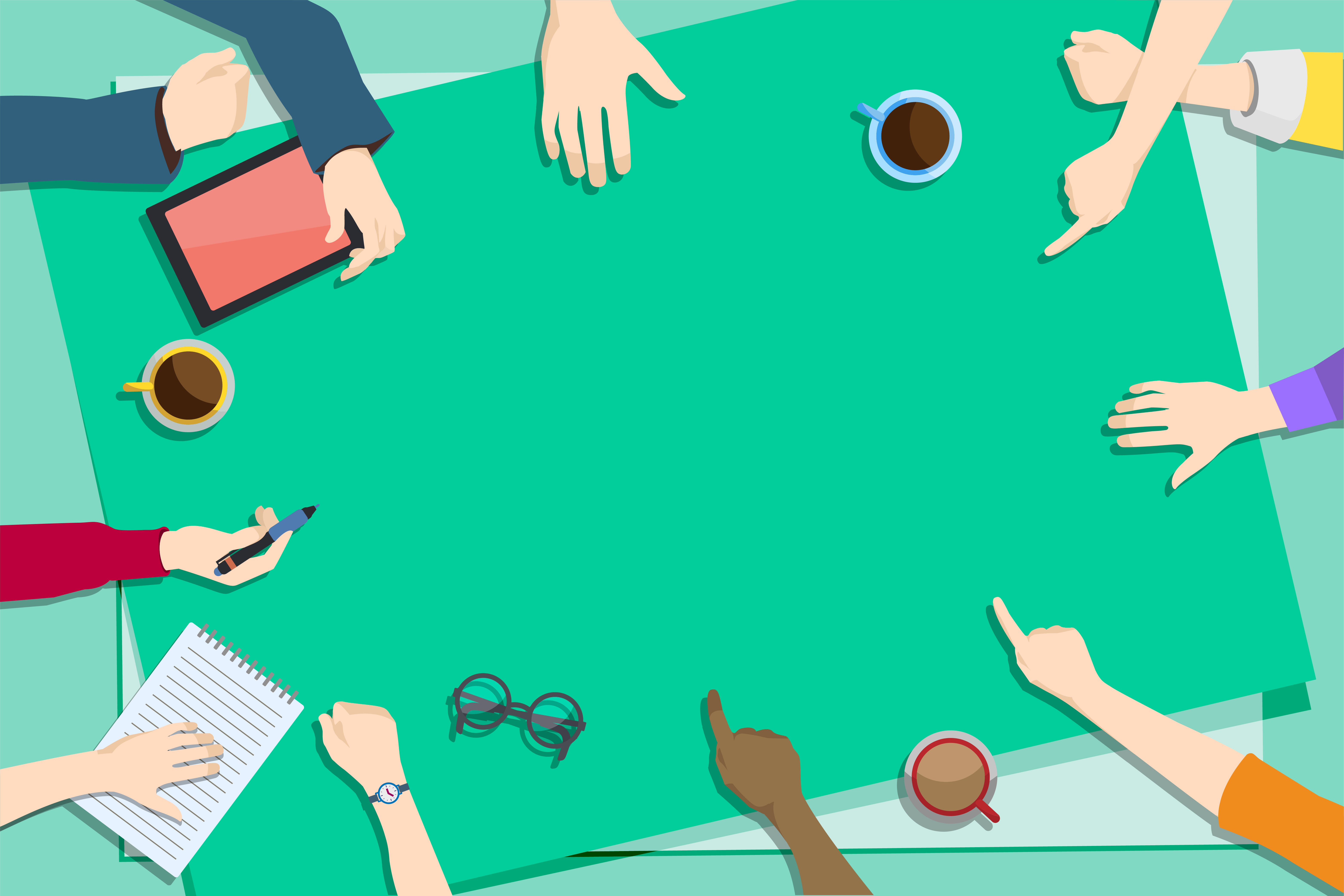
© Image by rawpixel.com on Freepik
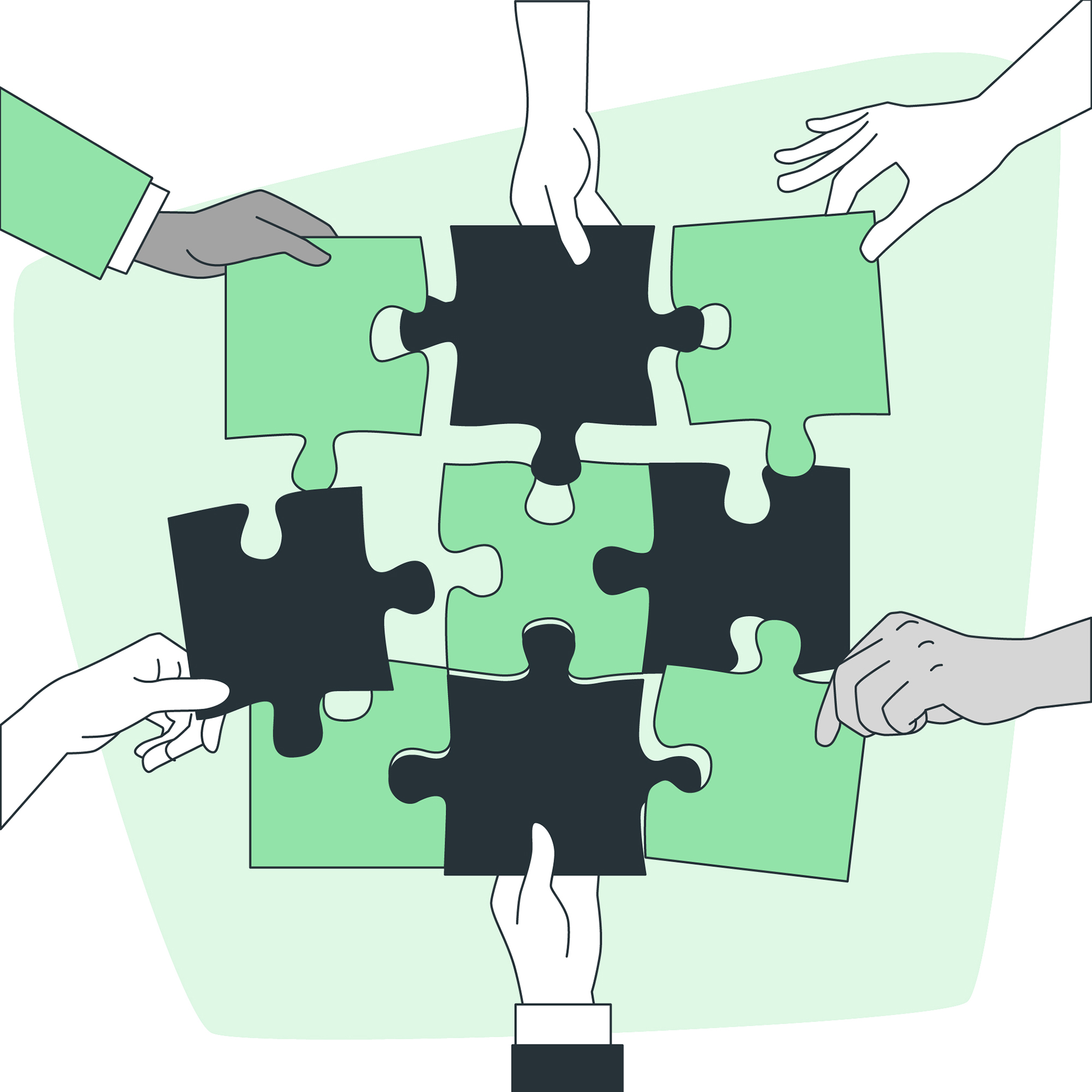
© Image by rawpixel.com on Freepik
Contact
Peter Krawitz
Director
© None
How to find us:
Approach option 1:
- BAB 565 to the Bonn-Poppelsdorf exit.
- The exit leads directly onto Reuterstraße, towards Bad Godesberg
- 1st traffic light turn right into Jagdweg (from here sign “Uni-Kliniken”)
- straight towards the Johannesschule.
- at the school left up the hill.
- At the next intersection turn right and then follow the street.
- Parking lot: in front of the main gate (entry only possible in exceptional cases)
Access option 2:
- B 42 / CHAPTER 562
- over the south bridge (Konrad Adenauer Bridge) B9 towards Bonn city center
- At Bundeskanzlerplatz keep left (direction BAB / from here sign "Uni-Kliniken")
- 2nd option on the left (into Hausdorffstrasse)
- 2nd traffic light on the right (into Eduard-Otto Straße)
- At the roundabout take the 3rd exit (into Graf-Stauffenberg Str.) then follow the street
- Parking lot: in front of the main gate (entry only possible in exceptional cases)
- In the main station follow the signs "Busbahnhof"
- From bus platform A1, Bus line 601/600 in the direction of "Uniklinik Süd", "Ippendorf Altenheim"
- Get down at "Bonn Uniklinikum Nord"
- Timetable VRS: Click here to get more information